Plotly Dash (The official name seems to be Dash, but since there are various other apps named Dash, I will use this notation) is a frame of a Python web application. It is a work. There are many Python web frameworks such as Flask and Bottle, but Plotly Dash can incorporate visualized data using Plotly.
Install with pip. I have not confirmed whether it can be installed with conda.
pip install dash==0.17.7 # The core dash backend
pip install dash-renderer==0.7.4 # The dash front-end
pip install dash-html-components==0.7.0 # HTML components
pip install dash-core-components==0.11.0 # Supercharged components
pip install plotly==2.0.12 # Plotly graphing library used in examples
import dash
import dash_core_components as dcc
import dash_html_components as html
import numpy as np
x = np.linspace(-np.pi, np.pi, 10)
y = np.sin(x)
app = dash.Dash()
app.layout = html.Div(children=[
html.H1(children='H1 text'),
html.Div(children='''
div text
'''),
dcc.Graph(
id='example-graph',
figure={
'data': [
{'x': x, 'y': np.sin(x), 'type': 'line', 'name': 'line'},
{'x': x, 'y': np.cos(x), 'type': 'bar', 'name': 'bar'},
],
'layout': {
'title': 'Graph title'
}
}
)
])
if __name__ == '__main__':
app.run_server(debug=True)
You don't have to write HTML as above, you can just do the Python code. Plotly is responsible for drawing the graph.
Markdown It supports Markdown notation.
import dash
import dash_html_components as html
import dash_core_components as dcc
markdown_doc = dcc.Markdown('''
#Heading 1
##Heading 2
###Heading 3
---
*Bullets
*Bullets
---
[Rinku](http://commonmark.org/help)
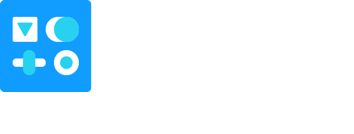
>Quote
''')
app = dash.Dash()
app.layout = html.Div(markdown_doc)
if __name__ == '__main__':
app.run_server(debug=True)
I couldn't express the table in my environment. Please let me know if anyone has succeeded.
Live Updates It also supports dynamic graphs.
The example below uses psutil
to monitor the resource status of the machine in real time.
import dash
from dash.dependencies import Input, Output, Event
import dash_core_components as dcc
import dash_html_components as html
import datetime
import plotly
import numpy as np
import pandas as pd
import psutil
colors = {
'background': '#111111',
'text': '#7FDBFF'
}
app = dash.Dash(__name__)
app.layout = html.Div(children=[
html.Div([
html.H4('Oreore system monitor'),
html.Div(id='live-update-text'),
dcc.Graph(id='live-update-graph'),
html.H4('Process list'),
html.Div(id='live-update-proc'),
dcc.Interval(
id='interval-component',
interval=1 * 1000 # in milliseconds
)
])
],
style={'backgroundColor': colors['background'], 'color': colors['text']}
)
class Context:
def __init__(self):
self.t = []
self.cpu = []
self.per_cpu = [[] for x in range(psutil.cpu_count())]
self.mem = []
@classmethod
def append_data(cls, d1, d2):
n = len(d1)
if n > 100:
del d1[0:n - 99]
d1.append(d2)
context = Context()
# The `dcc.Interval` component emits an event called "interval"
# every `interval` number of milliseconds.
# Subscribe to this event with the `events` argument of `app.callback`
@app.callback(Output('live-update-text', 'children'),
events=[Event('interval-component', 'interval')])
def update_metrics():
now = datetime.datetime.now()
hour, minute, second = now.hour, now.minute, now.second
style = {'padding': '5px', 'fontSize': '16px'}
return [
html.Span('CPU: {}%'.format(context.cpu[-1]), style=style),
html.Span('Memory: {}%'.format(context.mem[-1]), style=style)
]
# Multiple components can update everytime interval gets fired.
@app.callback(Output('live-update-graph', 'figure'),
events=[Event('interval-component', 'interval')])
def update_graph_live():
# global context
context.append_data(context.t, datetime.datetime.now())
context.append_data(context.cpu, psutil.cpu_percent())
for data, pct in zip(context.per_cpu, psutil.cpu_percent(percpu=True)):
context.append_data(data, pct)
context.append_data(context.mem, psutil.virtual_memory().percent)
# Create the graph with subplots
fig = plotly.tools.make_subplots(rows=2, cols=1, vertical_spacing=0.2)
fig['layout']['margin'] = {
'l': 30, 'r': 10, 'b': 30, 't': 10
}
fig['layout']['plot_bgcolor'] = colors['background']
fig['layout']['paper_bgcolor'] = colors['background']
fig['layout']['font'] = {'color': colors['text']}
fig['layout']['legend'] = {'x': 0, 'y': 1, 'xanchor': 'left'}
fig['layout']['yaxis1'].update(range=[0, 100])
fig['layout']['yaxis2'].update(range=[0, 100])
fig.append_trace({
'x': context.t,
'y': context.cpu,
'name': 'cpu',
'mode': 'lines',
'type': 'scatter',
}, 1, 1)
for i, y in enumerate(context.per_cpu):
fig.append_trace({
'x': context.t,
'y': y,
'name': 'cpu {}'.format(i),
'mode': 'lines',
'type': 'scatter',
}, 1, 1)
fig.append_trace({
'x': context.t,
'y': context.mem,
'name': 'memory',
'mode': 'lines',
'type': 'scatter',
'fill': 'tonexty',
}, 2, 1)
return fig
def get_proc_df():
def get_proc(proc):
try:
pinfo = proc
except psutil.NoSuchProcess:
pass
return (pinfo.pid, pinfo.name(), pinfo.memory_percent(), pinfo.cpu_percent())
data = [get_proc(proc) for proc in psutil.process_iter()]
df = pd.DataFrame(data, columns=['pid', 'name', 'memory', 'cpu'])
df['memory'] = df['memory'].map(lambda x: '{:.2f}%'.format(x))
df['cpu'] = df['cpu'] / psutil.cpu_count()
df['cpu'] = df['cpu'].map(lambda x: '{:.2f}%'.format(x))
return df.sort_values('cpu', ascending=False)
@app.callback(Output('live-update-proc', 'children'),
events=[Event('interval-component', 'interval')])
def generate_table():
df = get_proc_df()
max_rows = 10
return html.Table(
# Header
[html.Tr([html.Th(col) for col in df.columns])] +
# Body
[html.Tr([
html.Td(df.iloc[i][col], style={'width': '8em'}) for col in df.columns
]) for i in range(min(len(df), max_rows))]
)
if __name__ == '__main__':
app.run_server(debug=True)
You can interactively control the output contents while operating the UI. I will write how to do it soon.
Recommended Posts