Plotly Dash (Der offizielle Name scheint Dash zu sein, aber da es verschiedene andere Apps mit dem Namen Dash gibt, werde ich diese Notation verwenden) ist ein Frame einer Webanwendung von Python. Es ist eine Arbeit. Es gibt viele Python-Webframeworks wie Flask und Bottle, aber Plotly Dash kann mithilfe von Plotly visualisierte Daten einbinden.
Mit pip installieren. Ich habe nicht bestätigt, ob es mit conda installiert werden kann.
pip install dash==0.17.7 # The core dash backend
pip install dash-renderer==0.7.4 # The dash front-end
pip install dash-html-components==0.7.0 # HTML components
pip install dash-core-components==0.11.0 # Supercharged components
pip install plotly==2.0.12 # Plotly graphing library used in examples
import dash
import dash_core_components as dcc
import dash_html_components as html
import numpy as np
x = np.linspace(-np.pi, np.pi, 10)
y = np.sin(x)
app = dash.Dash()
app.layout = html.Div(children=[
html.H1(children='H1 Text'),
html.Div(children='''
div Text
'''),
dcc.Graph(
id='example-graph',
figure={
'data': [
{'x': x, 'y': np.sin(x), 'type': 'line', 'name': 'line'},
{'x': x, 'y': np.cos(x), 'type': 'bar', 'name': 'bar'},
],
'layout': {
'title': 'Graphentitel'
}
}
)
])
if __name__ == '__main__':
app.run_server(debug=True)
Sie müssen nicht wie oben HTML schreiben, sondern nur Python-Code. Plotly ist für das Zeichnen des Diagramms verantwortlich.
Markdown Es unterstützt die Markdown-Notation.
import dash
import dash_html_components as html
import dash_core_components as dcc
markdown_doc = dcc.Markdown('''
#Überschrift 1
##Überschrift 2
###Überschrift 3
---
*Kugel
*Kugel
---
[Rinku](http://commonmark.org/help)
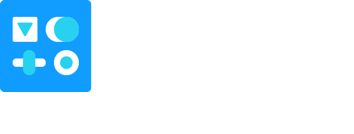
>Zitat
''')
app = dash.Dash()
app.layout = html.Div(markdown_doc)
if __name__ == '__main__':
app.run_server(debug=True)
Ich konnte den Tisch in meiner Umgebung nicht ausdrücken. Bitte lassen Sie mich wissen, wenn jemand erfolgreich war.
Live Updates Es unterstützt auch dynamische Diagramme.
Im folgenden Beispiel wird "psutil" verwendet, um den Ressourcenstatus einer Maschine in Echtzeit zu überwachen.
import dash
from dash.dependencies import Input, Output, Event
import dash_core_components as dcc
import dash_html_components as html
import datetime
import plotly
import numpy as np
import pandas as pd
import psutil
colors = {
'background': '#111111',
'text': '#7FDBFF'
}
app = dash.Dash(__name__)
app.layout = html.Div(children=[
html.Div([
html.H4('Oreore Systemmonitor'),
html.Div(id='live-update-text'),
dcc.Graph(id='live-update-graph'),
html.H4('Prozessliste'),
html.Div(id='live-update-proc'),
dcc.Interval(
id='interval-component',
interval=1 * 1000 # in milliseconds
)
])
],
style={'backgroundColor': colors['background'], 'color': colors['text']}
)
class Context:
def __init__(self):
self.t = []
self.cpu = []
self.per_cpu = [[] for x in range(psutil.cpu_count())]
self.mem = []
@classmethod
def append_data(cls, d1, d2):
n = len(d1)
if n > 100:
del d1[0:n - 99]
d1.append(d2)
context = Context()
# The `dcc.Interval` component emits an event called "interval"
# every `interval` number of milliseconds.
# Subscribe to this event with the `events` argument of `app.callback`
@app.callback(Output('live-update-text', 'children'),
events=[Event('interval-component', 'interval')])
def update_metrics():
now = datetime.datetime.now()
hour, minute, second = now.hour, now.minute, now.second
style = {'padding': '5px', 'fontSize': '16px'}
return [
html.Span('CPU: {}%'.format(context.cpu[-1]), style=style),
html.Span('Memory: {}%'.format(context.mem[-1]), style=style)
]
# Multiple components can update everytime interval gets fired.
@app.callback(Output('live-update-graph', 'figure'),
events=[Event('interval-component', 'interval')])
def update_graph_live():
# global context
context.append_data(context.t, datetime.datetime.now())
context.append_data(context.cpu, psutil.cpu_percent())
for data, pct in zip(context.per_cpu, psutil.cpu_percent(percpu=True)):
context.append_data(data, pct)
context.append_data(context.mem, psutil.virtual_memory().percent)
# Create the graph with subplots
fig = plotly.tools.make_subplots(rows=2, cols=1, vertical_spacing=0.2)
fig['layout']['margin'] = {
'l': 30, 'r': 10, 'b': 30, 't': 10
}
fig['layout']['plot_bgcolor'] = colors['background']
fig['layout']['paper_bgcolor'] = colors['background']
fig['layout']['font'] = {'color': colors['text']}
fig['layout']['legend'] = {'x': 0, 'y': 1, 'xanchor': 'left'}
fig['layout']['yaxis1'].update(range=[0, 100])
fig['layout']['yaxis2'].update(range=[0, 100])
fig.append_trace({
'x': context.t,
'y': context.cpu,
'name': 'cpu',
'mode': 'lines',
'type': 'scatter',
}, 1, 1)
for i, y in enumerate(context.per_cpu):
fig.append_trace({
'x': context.t,
'y': y,
'name': 'cpu {}'.format(i),
'mode': 'lines',
'type': 'scatter',
}, 1, 1)
fig.append_trace({
'x': context.t,
'y': context.mem,
'name': 'memory',
'mode': 'lines',
'type': 'scatter',
'fill': 'tonexty',
}, 2, 1)
return fig
def get_proc_df():
def get_proc(proc):
try:
pinfo = proc
except psutil.NoSuchProcess:
pass
return (pinfo.pid, pinfo.name(), pinfo.memory_percent(), pinfo.cpu_percent())
data = [get_proc(proc) for proc in psutil.process_iter()]
df = pd.DataFrame(data, columns=['pid', 'name', 'memory', 'cpu'])
df['memory'] = df['memory'].map(lambda x: '{:.2f}%'.format(x))
df['cpu'] = df['cpu'] / psutil.cpu_count()
df['cpu'] = df['cpu'].map(lambda x: '{:.2f}%'.format(x))
return df.sort_values('cpu', ascending=False)
@app.callback(Output('live-update-proc', 'children'),
events=[Event('interval-component', 'interval')])
def generate_table():
df = get_proc_df()
max_rows = 10
return html.Table(
# Header
[html.Tr([html.Th(col) for col in df.columns])] +
# Body
[html.Tr([
html.Td(df.iloc[i][col], style={'width': '8em'}) for col in df.columns
]) for i in range(min(len(df), max_rows))]
)
if __name__ == '__main__':
app.run_server(debug=True)
Sie können den Ausgabeinhalt interaktiv steuern, während Sie die Benutzeroberfläche bedienen. Ich werde bald schreiben, wie es geht.